Learn how robust frameworks and high-quality data are essential for reliable AI outcomes, especially in sensitive sectors like healthcare and finance.
As artificial intelligence surges, so too does the importance of data. Clean, stable data is what feeds the algorithms, forms the outcomes, and ultimately, dictates the success or failure of AI initiatives. And yet, amongst the excitement of increasingly available AI applications, the essential elements of data governance and quality often take a back seat.
Data governance is the framework that ensures data is managed, utilized, and protected effectively. It encompasses policies, procedures, and controls to enforce data integrity, privacy, and compliance. Without robust governance processes in place, AI initiatives take the risk of operating incomplete or biased data, which can easily lead to inaccurate conclusions and high consequential probabilities.
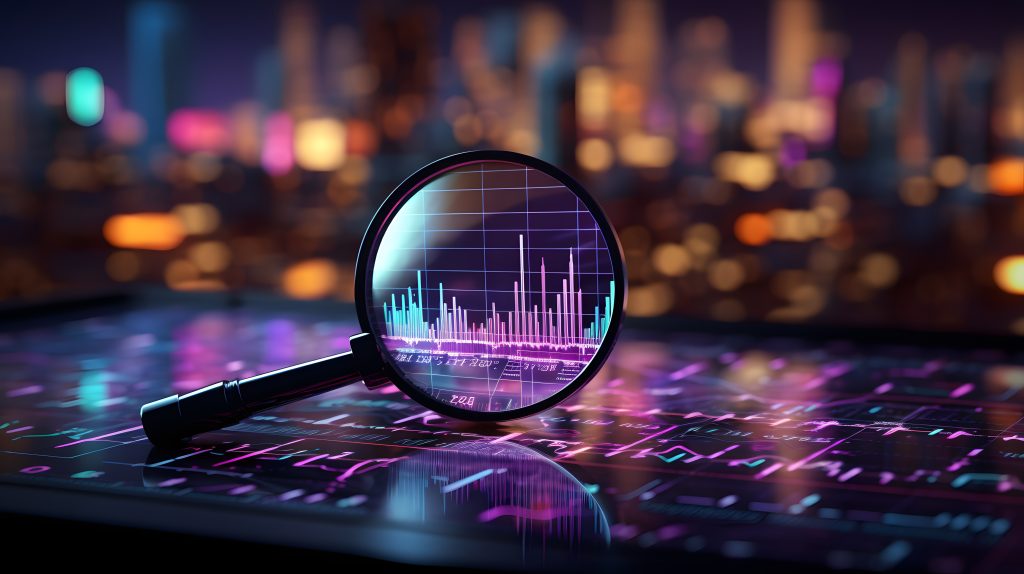
Similarly, data quality is vital. Garbage in, garbage out—a common mantra within the world of data science. High-quality data is accurate, consistent, and reliable, laying the foundation for AI models to deliver meaningful insights and predictions. Poor data quality, on the other hand, introduces noise and uncertainty, undermining the credibility and usefulness of AI-driven solutions.
Take for a second, a scenario where a healthcare provider institutes AI to aid in diagnosing diseases. Without data governance, there’s a risk of unauthorized access to sensitive patient information, which in turn, violates privacy policies and breaks down trust throughout the organization. Furthermore, if the data feeding into the AI model is incomplete or contains inaccurate values, the diagnoses generated could be misleading, putting patient health at stake.
“Without data governance, there’s a risk of unauthorized access to sensitive patient information, which in turn, violates privacy policies and breaks down trust throughout the organization.”
Also, in the financial industry, AI algorithms can drive decisions ranging from credit approvals to investment strategies. Inaccurate or biased data can lead to discriminatory outcomes and expose organizations to legal liabilities.
To harness the full potential of AI and mitigate risks, businesses must prioritize data governance and quality initiatives. This involves establishing clear accountability for data management, implement quality assurance processes, and adopt a culture of data stewardship across the organization.
In summary, the success of AI hinges on the integrity and reliability of the data it operates on. By prioritizing data governance and quality, organizations can unlock the true transformative power of artificial intelligence while upholding ethical standards and regulatory compliance. It’s not just about the algorithms—it’s about the data that fuels them.
Written By:
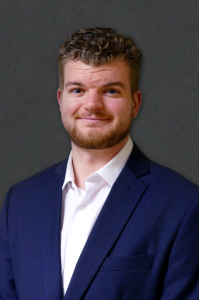
Cody Krietemeyer
Practice Leader, Data Engineering
Data Strategy Group